Data Quality Management: Ultimate Guide To Improve your Data Quality
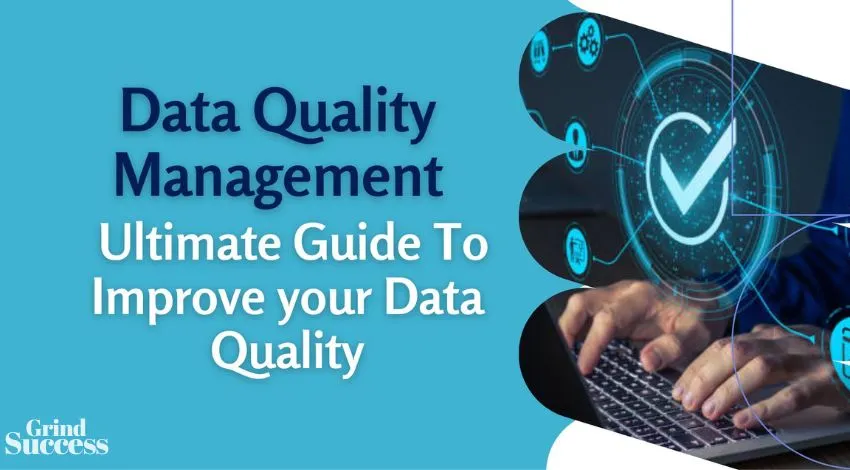
Data quality management, or DQM, is a technique used by data organizations to maintain the quality of data. In another word, DQM helps to keep the data clean from any errors, keep it up-to-date and well-managed, and ready to use by the experts for further process.
High-quality data helps in the company’s analytical activities including better decision-making, improving conversion rates, enhancing customer satisfaction, and many more. Hence, it is necessary for a company to focus on data quality management.
Since every company has different demands and standards for data quality, there are differences in data quality management among enterprises.
These rely on several factors, including the company’s size, the size of the dataset, the sources used, the types of individuals you need to oversee the data quality, the parameters you need to use to gauge it, and the procedures you must set up.
In this blog, we’ve compiled six such practices that any company, irrespective of their size, source, and type can perform to maintain a good quality of data.
Table of Contents
Why Do Businesses Need Data Quality Management?
While the digital age has effectively stimulated innovation worldwide, it has also facilitated what is known as the “data problem” — low-quality data in such large quantities that it is difficult for businesses to comprehend and, in some cases, is worthless.
As a result, data quality management becomes an essential procedure for making sense of such vast quantities of data. It aims to help businesses find data issues that need to be fixed.
Additionally, it helps to check if the systems’ data are accurate enough to carry out any desired purposes.
What are the Metrics that Measure Data Quality?
Metrics for measuring data quality are crucial for evaluating your efforts to improve the quality of your data. Hence, metrics should be clearly specified. Here are some of them.
Data Quality Management: 6 Best Practices
1. Implement a Single Source of Truth
Since data drives many crucial business decisions, it’s crucial that all business divisions settle on a single source of precise, high-quality data. To do so, businesses can adopt the concept of a single source of truth.
SSOT guarantees that every employee in an organization bases business choices on the same reliable and accurate facts.
Once the SSOT is acknowledged by the whole company as the source of reliable data, that data may be kept up to date in accordance with the organization’s data quality criteria and used by anybody for any objective to provide reliable business insights.
2. Draw Meaningful Insights From Issues
If you find low-quality data in your company’s database, you don’t need to delete all of your data. Instead, you might gain insights from the poor data that could help you refine your operations in the future.
In this case, it would be prudent to look over the available data, pinpoint the cause of the quality issues, and fix them. By doing so, the groundwork for dealing with clean, high-quality data will be laid, and common problems that may be avoided or fixed in the future will also be identified.
3. Evaluate Data On a Regular Basis
This entails thoroughly examining the company’s data repositories to identify critical problems with the system’s data quality. A thorough, impartial data evaluation is crucial to determine how bad data undermines corporate objectives.
Additionally, it offers a benchmark against which to invest, plan, and evaluate data quality upgrades and track subsequent adjustments’ effects.
4. Perform Data Governance
An organization’s ability to use data effectively and safely is ensured by a collection of procedures, responsibilities, standards, and measurements known as data governance.
A governance system must be implemented to ensure that roles and responsibilities for data quality management are clearly defined.
5. Invest in Automation Tools
Manual data entry is one of the main contributors to poor data quality because of the high probability of human error. This risk grows when a business needs a lot of personnel to enter data.
To avoid this, investing in automation tools for the data entry process is a good idea. Furthermore, these tools can be customized to your integrations and policies to ensure that your data is accurate everywhere.
6. Create a Data Dictionary
Making a data dictionary part of your governance plan is a good idea. This should include an accessible, user-friendly glossary of pertinent terms to characterize the firm data. By doing this, you can guarantee that data definitions are applied consistently throughout the organization.
Conclusion
Business executives recognize the value of data since it is used in all facets of operations, from simple tasks to sophisticated business analytics.
However, due to duplication of effort, a lack of data knowledge, and erroneous results, most data teams end up spending more time than necessary. All of these issues result from poor or nonexistent data quality management.
To address your data quality management requirements, you have to adopt some quality measures.
However, if you find it to be too complex, there are other various options in the market. You can hire data management outsourcing services from a reputed company. These data experts can improve the quality of your data and keep them up-to-date.
Author Bio:
Gracie Ben is a data analyst currently working at DataEntryIndia., a leading company providing data entry & mining services & other data-related solutions.
For more than ten years, she has actively contributed to the growth of many enterprises & businesses (startups, SMEs, and big companies) by guiding them to utilize their data assets.
Having a keen interest in data science, Gracie keeps herself up-to-date on all the latest data trends and technologies shaping the industry and transforming businesses.
She has written over 1600 articles and informative blogs so far covering various topics, including data entry, data management, data mining, web research, and more.
This post was proofread by Grammarly. Try it – It’s Free!
Millions trust Grammarly’s free writing app to make their online writing clear and effective. Getting started is simple
Email, SMS, and more — Klaviyo brings your marketing all together, fueling growth without burning through time and resources.
Deliver more relevant email and text messages — powered by your data. Klaviyo helps you turn one-time buyers into repeat customers with all the power of an enterprise solution and none of the complexity.