Image Annotation in [2024]: Everything You Need to Know
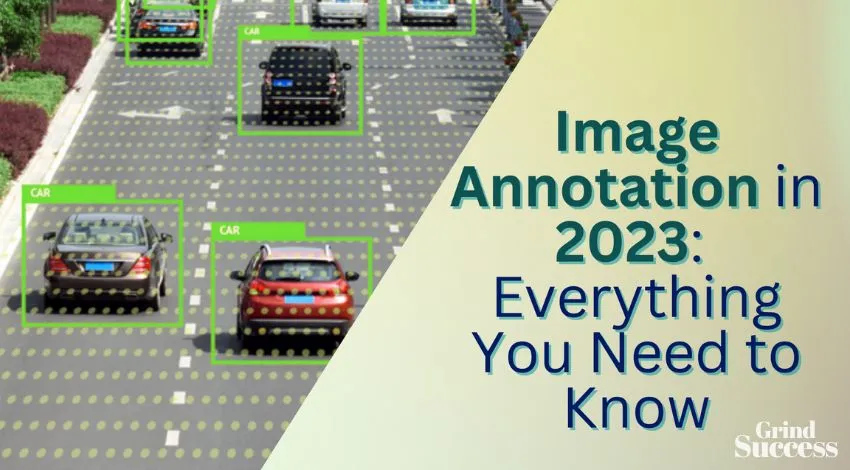
Computer Vision is a crucial subcategory of AI that allows machines to mimic human behavior (to some extent) and make decisions independently via visual data analysis and interpretation.
Some major examples of AI-based models using it in the real world include facial recognition, self-driving cars, automated traffic controls, unmanned drones, and more.
To achieve such results, most computer vision models are trained to better understand and interpret information from images, videos, or other different types of visual inputs through image and video annotation.
This blog covers the overview of image annotation, its importance, benefits, use cases, and how expert image annotation services can be advantageous to your business in [2024].
Let’s get started!
Image Annotation: Introduction
Enterprise data is a valuable asset that is present in multiple formats like audio, text, video, image, etc. Image annotation uses the images for training AI models by labeling the important aspects of it with predetermined criteria and tags.
This process helps alienate the target subject in the image with that of its background and other objects. It is this differentiation that helps Machine Learning algorithms to identify and learn about the target subject’s characteristics, which ultimately enables the resultant AI model to identify it in a random data sample.
The initial data sample set used for the training is called the training sample. It is high in number as these algorithms require multiple iterations of labeled data analysis to accurately identify the intended subject. Images are also annotated to be used for testing purposes to check if the algorithm is accurate enough for the task.
Besides the image and video data available within a company’s data servers, additional training and testing data may be used from external data sources like Microsoft’s COCO image library for data annotation that can contain hundreds of thousands to millions of samples.
Due to the sheer volume of data to be used and the complexity of this process, it is common practice for businesses to outsource the task to professional image annotation service providers.
The Importance of Image Annotation
The advantages and significance of image annotation are widely acknowledged and emphasized in business decisions. As per recent estimations, the market for AI and machine learning data solutions will reach $1.2 billion by [2024].
![Image Annotation in [2024]: Everything You Need to Know Image Annotation](https://grindsuccess.com/wp-content/uploads/2023/02/Image-Annotation.webp)
![Image Annotation in [2024]: Everything You Need to Know Image Annotation](https://grindsuccess.com/wp-content/uploads/2023/02/Image-Annotation.webp)
Such is the value that this frontier is capable of, and it’s just getting started. And backing it all up will be annotations of various data types. No aspect of business will be left untouched by image annotation, offering multiple benefits.
1. Faster Image-dependent Operations
Speed is critical for any business, and image//video-based operations can consume crucial time when done manually. Using AI that’s been developed via image annotation can speed up all those processes, saving precious time. This increases efficiency and productivity.
2. Increase in Enterprise Data Value
Every operation that is performed on enterprise data to improve its quality increases its value. And so does every use-case for it.
Image annotation requires high-quality enterprise data to be successful and expands the scope of that data by including it in new operations that it may not have been used for otherwise. Thus, the value of the stored enterprise visual data goes up, increasing its ROI.
3. Faster AI Development
Professionals tend to combine multiple types of annotation into a cohesive model that can better tackle the challenges of its use case.
If you have annotated images in your database, and you want to proceed with developing AI for another use case that requires image annotation along with other types of data annotation, then you’re well set for it. This is an aspect that expert data labeling service providers implement on a large scale.
Having annotated images in store can also help develop automated image annotation via deep learning, as they will serve as the initial data feed for the algorithms.
4. Maintenance of Market Position/Lead
How well a company does in the market today and in the years ahead depends on how it adopts AI and ML technology and adapts to changing market demands these technologies enable. Consolidating your brand’s market foothold is, therefore, enhanced by using Image annotation.
By using it for creating AIs that benefit your customers/clients, you send the message to them that you’re innovating and can deliver on your promises going ahead. This increases your chances of procuring long-term business from them, thus improving your brand image and possibly giving you a market leader if you don’t have one already.
5. More Skilled Staff
If you’re conducting image annotation in-house, then you’re likely to retrain your present employees besides hiring new ones. This act improves their overall skillset, making them more valuable to the company, especially in the coming age of more AI usage.
Even if you choose to go with an external image/video annotation services provider, you’ll have to assign staff to coordinate with that agency, thereby improving their skills in the process.
6. Reduction in Expenses/Losses
Losses can occur when poor-quality data is used for various operations. High-quality image annotation prevents that by ensuring the annotated data is well-managed and ready to be used whenever required.
It also reduces expenses that go toward generating such data as there are many free, open-source databases you could gain such data from for this purpose, some of which may even be annotated already.
Different Types of Image Annotation
There are three main types of image annotation that help in generating quality training datasets for computer vision models-
1. Image Classification
Preparing images for classification is also known as image tagging. Image classification aims to simply identify the presence of a particular object and name its predefined class.
An example is where multiple animals are “detected” in input images. Here, the annotator will possess a dataset of images with different animals that are predefined, and they will classify each image in the training set respectively. Thus, an animal’s species becomes its class and its image the input.
2. Object Detection
Object detection and recognition is the process of labeling objects based on location, shape, and the total number of objects available in an image. For example- annotators draw bounding boxes around entities like cars and other vehicles and pedestrians within traffic images.
With polygons, lines are drawn using dots placed on the outer edges of the object. The space thus formed within the bounds of the connected dots is then annotated using one of the predetermined sets of classes. This method can even be extended to isolate objects in individual frames of videos during video annotation.
3. Image Segmentation
Image segmentation is one of the essential processes for machine learning models. Each pixel of an image gets allocated a specific class, which is necessary for projects with high accuracy demands.
It is generally of three types:
Use Cases of Image Annotation across Industries
The Benefits of Choosing Data Labeling and Image Annotation Services in [2024]
The need to hire experts and outsource data labeling services and image annotation is growing as it resolves multiple challenges associated with annotation tasks. Have a look-
Time and Cost Savings
When you need to label and tag images manually, a lot of time is consumed. As mentioned above, machine learning requires a lot of high-quality datasets for training. Considering this, companies are looking to outsource their annotation and data labeling workloads to save on costs and time.
Reduced Errors and Complexities
Machine learning requires precise and error-free annotated data to run models effectively. If there is any error or misinterpretation in the image tagging, identifying, and labeling of the image, it may affect the whole training.
Therefore, it is considered prudent to hire image, audio, and text annotation service experts to be assured of accurate and high-quality annotated data.
Easy Access to Advanced Domain Expertise
Image tagging usually requires advanced domain knowledge and the right expertise. If it is overlooked, it may hamper the result. To annotate images and videos correctly, you need to ensure that the person holds considerable experience in preparing datasets for AI/ML models.
This way, you can also ensure that the process runs smoothly and without delay. Outsourcing offers such expertise the moment you hire an agency, instead of searching for it yourself and incurring delays, and the consequent losses.
Final Words
Hopefully, these insights helped you understand the concept of image annotation. Currently, AI-powered experiences are in demand, and businesses are heavily interested in image and video annotation processes.
However, you should always ensure that data annotation should be done by a team of skilled professionals with a deep understanding of what they’re annotating. Regardless of any image annotation type or the use case, a trusted image annotation service partner will bring tremendous value and efficiency to your next machine learning project.
Author Bio:
Jessica is a Content Strategist, currently engaged at Data-Entry-India.com- a globally renowned data entry and management company -for over five years. She spends most of her time reading and writing about transformative data solutions, helping businesses to tap into their data assets and make the most out of them.
So far, she has written over 2000 articles on various data functions, including data entry, data processing, data management, data hygiene, and other related topics. Besides this, she also writes about eCommerce data solutions, helping businesses uncover rich insights and stay afloat amidst the transforming market landscapes.